

Basic data visualization free#
Charts built in Data Wrapper are meant to be embedded in a website.Ĭhart Blocks is an online visualization tool with a free plan that lets you build and host 30 charts at a time.
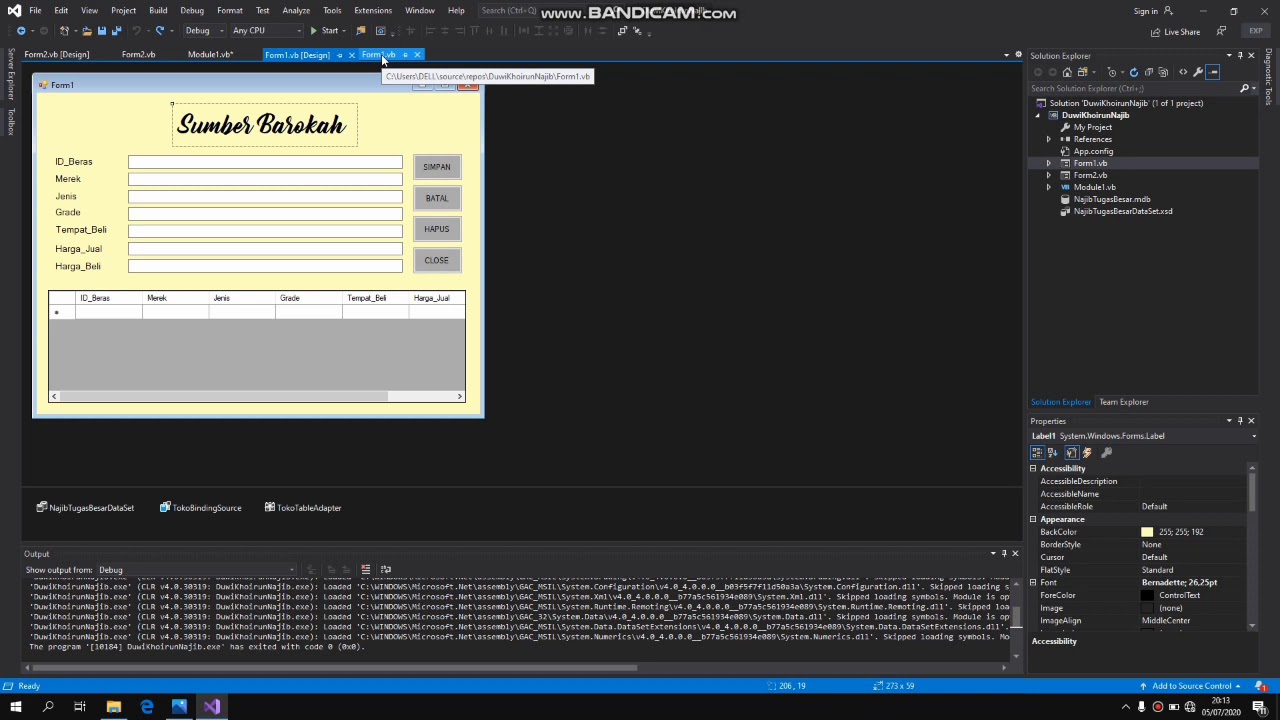
Instead of using default chart styles, users should take care to customize and refine their charts in Excel for best results.ĭata wrapper is a free, easy-to-use, web-based data visualization tool that allows only basic customizations but renders visually attractive graphics. Public visualizations should not use private or personally identifiable information.īecause it is included in Microsoft Office, Excel is a familiar tool to many people. As we can see, various options are there in the Column chart, and the requisite one should be selected. First, to build a column chart, select the data, and then select the required option from the Column chart option, as can be seen below. Publishing visualizations on the web requires a Tableau Public account. It is a very simple chart type that presents data in the form of vertical bars.
Basic data visualization license#
A free academic license is available to students, instructors, and non-profit academic researchers. Tableau produces a wide variety of beautiful interactive visualizations and maps, and its drag-and-drop interface makes it easy to learn and use.
Basic data visualization how to#
Finally, you may need to learn how to use a new software program depending on the results you want to achieve. In most cases, the dataset will also need to be cleaned and prepared a certain way in order for it to be compatible with the data visualization tool you want to use. Retrieved from īefore you begin a data visualization project, you will need to find a dataset to work with. In The Encyclopedia of Human-Computer Interaction, 2nd Ed. format_dict = #Simplified format dictionary with values that do make sense for our data df.head().style.format(format_dict).highlight_max(color='darkgreen').Few, S. To give an example of each type, I have added currency and percentage symbols even though they do not make any sense for this data. First, we define a format dictionary so that the numbers are shown in a legible way (with a certain number of decimals, date and hour in a relevant format, with a percentage, with a currency, …) Don’t panic, this is only a display and does not change the data, you will not have any problem to process it later. Using Pandas styles, we can get much more information when viewing the table. pd.set_option('display.max_rows', 500) pd.set_option('display.max_columns', 500) pd.set_option('display.width', 1000) Be careful with this option for big datasets, we can have problems showing them. With these commands, we increase the limits and we can visualize the whole data.
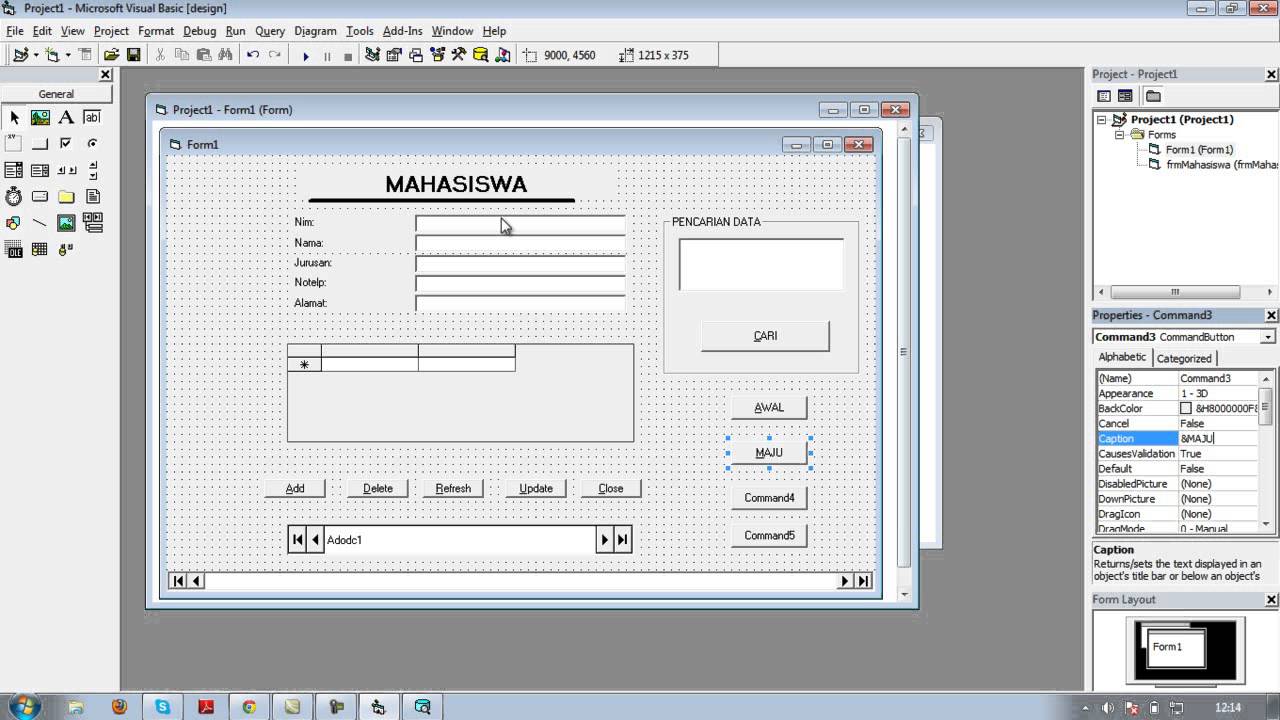
The first thing we must do is visualize a few examples to see what columns there are, what information they contain, how the values are coded… import pandas as pd df = pd.read_csv('temporal.csv') df.head(10) #View first 10 data rows We will simply use pandas to take a look at the data and get an idea of how it is distributed. Pandasīefore we move on to more complex methods, let’s start with the most basic way of visualizing data. We will use it in the last section of the article when working with maps. The file mapa.csv includes popularity data separated by country. In addition, I have added a categorical variable (ones and zeros) to demonstrate the functionality of charts with categorical variables. The first one we will use in the vast majority of the tutorial includes popularity data of the three terms over time (from 2004 to the present, 2020). There are two files temporal.csv and mapa.csv. They have been extracted from a famous search engine. They are data on the popularity of searches on the Internet for three terms related to artificial intelligence (data science, machine learning and deep learning). We will work with two datasets that will adapt to the visualizations we show in the article, the datasets can be downloaded here. We will start with the most basic visualization that is looking at the data directly, then we will move on to plotting charts and finally, we will make interactive charts. We will also see which library is recommended to use on each occasion and the unique capabilities of each library.

Let’s see the main libraries for data visualization with Python and all the types of charts that can be done with them. Complete Guide to Data Visualization with Python
